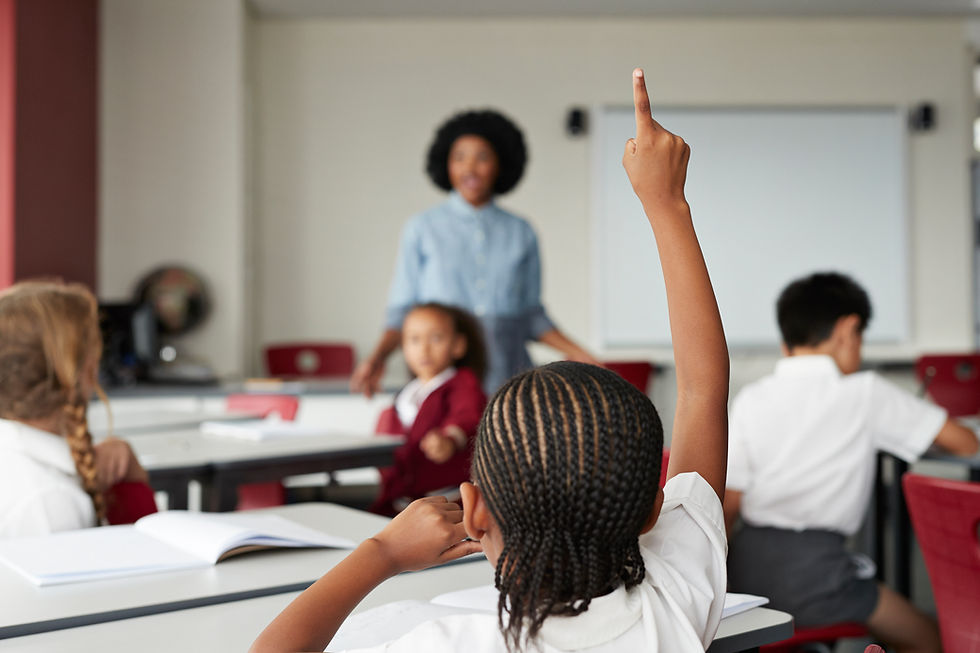
AI for Predictive analytics
An AI-driven system to predict the performances of the students at school.
AI for Predictive Analytics in Education
Overview: AI-powered predictive analytics can transform educational strategies by utilizing data-driven insights to enhance student performance, optimize learning paths, and improve institutional decision-making. By analyzing historical and real-time data, these systems can forecast trends, identify at-risk students, and provide actionable insights to educators and administrators.
Functionality:
Student Performance Prediction: AI models can analyze grades, attendance records, and engagement metrics to predict individual student performances and identify those who might be at risk of underperforming or dropping out.
Curriculum Optimization: Analyze academic results across different courses and student feedback to recommend changes to the curriculum, adapting teaching methods to better meet student needs.
Resource Allocation: Use predictive models to determine the optimal allocation of resources such as faculty, facilities, and financial aid, based on predicted student enrollment and course popularity.
Admission Predictions: Help admissions officers predict applicant success and cultural fit within the school environment, optimizing the selection process.
Implementation Steps in Education
Data Collection:
Gather extensive datasets from across the educational ecosystem, including student demographics, academic records, engagement metrics from learning platforms, and financial data.
Model Development and Training:
Develop predictive models using machine learning algorithms tailored to the educational sector. Train these models with collected data, continually refining them to improve accuracy and relevance.
Integration:
Integrate predictive analytics tools with existing educational IT systems, such as student information systems (SIS), learning management systems (LMS), and administrative platforms.
Testing and Iteration:
Conduct pilot testing with a controlled group of users to gauge the effectiveness of predictive insights. Use feedback to make iterative improvements to the models.
Full Deployment and Continuous Evaluation:
Deploy the system across the institution, using ongoing data collection and model updating to ensure the predictive analytics evolve with changing educational trends and needs.
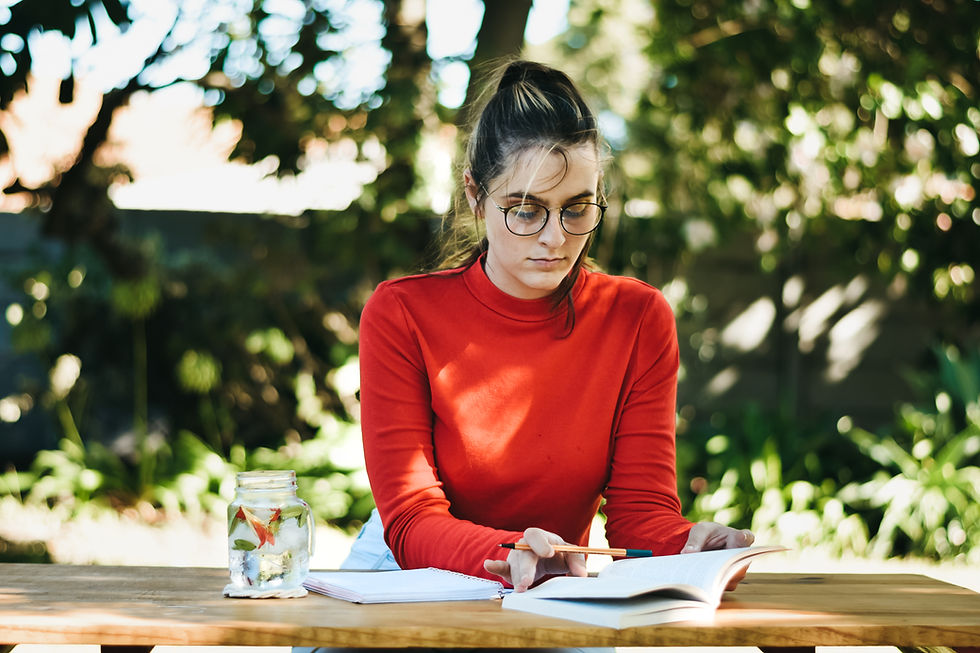
Case for Educational Institutions: Implementing Predictive Analytics at a University
Challenge:
Educational institutions often struggle with high dropout rates and student disengagement, alongside challenges in resource allocation and curriculum development.
Proposed Solution:
Implement an AI-driven predictive analytics system to proactively identify and address student needs, optimize academic programs, and efficiently allocate resources.
Expected Benefits:
Improved Student Retention and Performance: Early identification of at-risk students allows for timely interventions, improving retention rates and academic outcomes.
Enhanced Decision-Making: Data-driven insights assist in making informed decisions about curriculum changes, resource distribution, and admissions strategies.
Cost Efficiency: Better prediction of resource needs reduces waste and ensures that institutional resources are used effectively.
ROI Justification:
The potential increase in student retention and success rates can significantly enhance an institution's reputation and financial health, justifying the initial investment in AI technologies.
Project Gallery
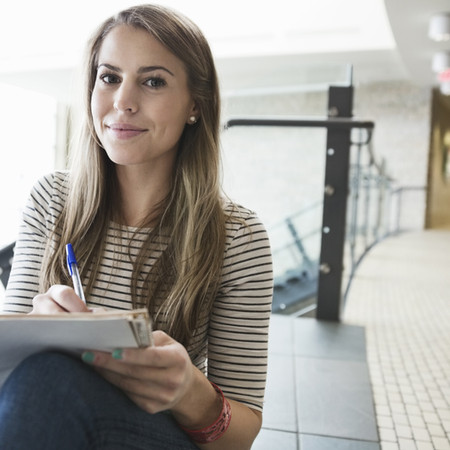
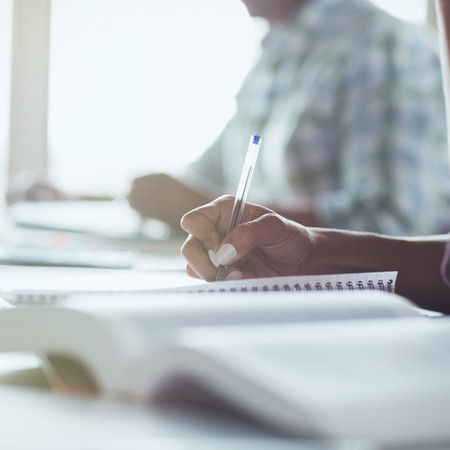
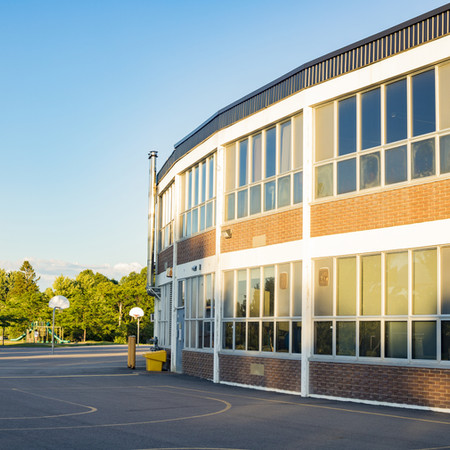